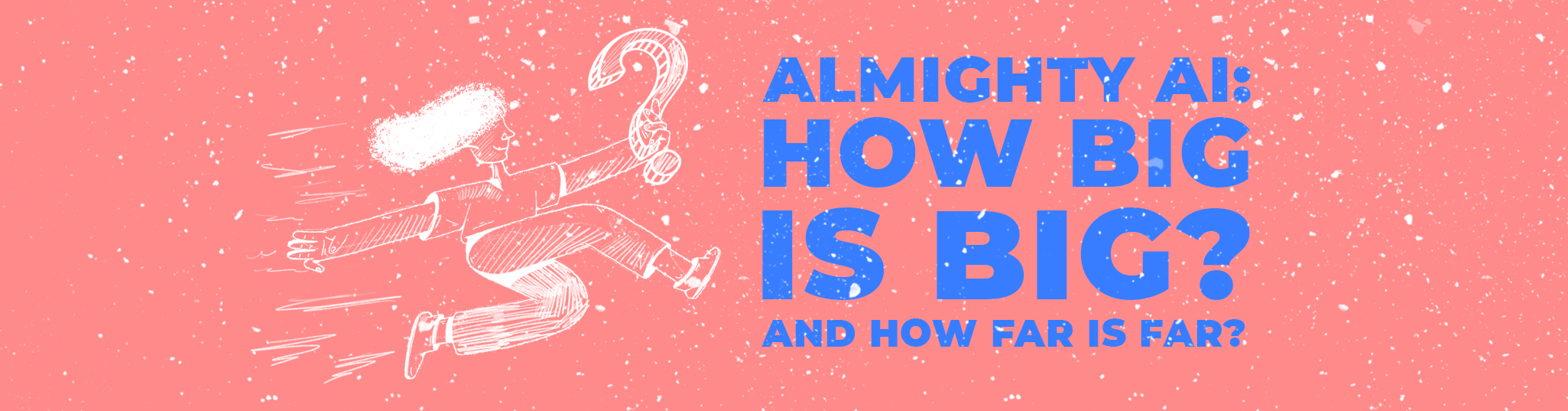
Almighty AI: How Big is Big? and How Far is Far?
The days of computational finance and basic algorithmic trading are behind us, and now automation has expanded from the trading floor to touch almost every aspect of a bank’s technology infrastructure. But how far will it go? Are we staring at an AI-fueled future, where everything from the branch to the back office is run through machine-made decisions? Or is the reality something more nuanced? What type of model will banks and financial services firms embrace as customers demand more data, more insights, and quicker service?
Artificial Intelligence (AI) has become sought-after knowledge as businesses are apt to learn in the growing kingdom of automation, machine learning, robotics, analytics and more. Financial services providers are making efforts to adopt AI for mundane task automation, consistent customer services, deep-dive behavior analysis, as well as efficient fraud finding. They also need to manage the risks and concerns that arise along the journey of transformation. As artificial intelligence (AI) significantly changes the traditional operating models of financial institutions—shifting strategic priorities and upending the competitive dynamics of the financial ecosystem—how can financial institutions better embrace AI and prepare themselves for the future?
World Economic Forum (Forum) and Deloitte Global’s latest report studies the strategic, operational, regulatory, and societal implications of AI on the financial services industry to elucidate previously sensationalized debates and help the industry look forward. Artificial intelligence is changing the physics of financial services, weakening the bonds that have held together with the component parts of incumbent financial institutions and opening the door to entirely new operating models.
Institutions will turn AI-enabled back-office operations into external services, both accelerating the rate at which these capabilities improve and necessitating others to become consumers of those capabilities to avoid falling behind Business leaders are thinking strategically around AI is for its potential to unlock new sources of revenue. Earlier, functions such as internal IT were seen as a cost center. In today’s world, due to the cost and competitive pressure, areas of the business which were traditionally considered to be cost centers are required to reinvent themselves into revenue and profit centers. The expectation of AI is no different. There is a need to justify the investments made in this technology – and find a way for it to unlock new streams of revenue in traditional organizations. Here are two key ways in which business leaders can monetize AI:
Indirect Monetization is one of the forms of leveraging AI to unlock new revenue streams. It involves embedding AI into traditional business processes with a focus on driving increased revenue. We hear of multiple companies from Amazon to Google that use AI-powered recommendation engines to drive incremental revenue through intelligent recommendations and smarter bundling. The action item for business leaders is to engage stakeholders across the enterprise to identify areas where AI can be deeply ingrained within tech properties to drive incremental revenue.
Direct Monetization involves directly adding AI as a feature to existing offerings. Examples abound in this area – from Salesforce bringing in Einstein into their platform as an AI-centric service to cloud infrastructure providers such as Amazon and Microsoft adding AI capabilities into their cloud offerings. Business leaders should brainstorm about how AI augments their core value proposition and how it can be added to their existing product stack.
As past methods of differentiation erode, AI presents an opportunity for institutions to escape a "race to the bottom" in price competition by introducing new ways to distinguish themselves from customers AI is real and it creates increased competitive intensity across brands. Traditionally, brands had two main differentiators or dimensions—cost and perceived quality.
Now, customer experience adds a third dimension or differentiator. Low cost and product quality won’t go far against competitors who transform their customer experience and delight their clients. AI impacts the customer service industry in multiple ways. And it breaks through the differentiation barrier on all three dimensions—cost, quality, and customer experience.
Future customer experiences will be centered around AI, which automates much of customers’ financial lives and improves their financial outcomes. What if our financial lives were driven by a self-driving wallet, leveraging data, algorithms and machine learning to navigate the twists and turns of our ever-changing needs and opportunities? Imagine a Siri-like assistant that is part accountant, part budgeting guru, part fortune teller, constantly monitoring and learning from your financial data in order to manage your money. Knowing how much you earn and owe, it would determine the optimal schedule for drawing down wages, paying bills, and saving for both emergencies and longer-term goals. It would constantly be on the lookout for opportunities to switch to cheaper or better-suited financial products, and it would warn you ahead of time about upcoming bumps in the road. This kind of modern financial advice is destined to become the new “free checking” of the banking industry in this digital era when checking accounts are indistinguishable from one provider to another, and a bank is less a place you go and more about what you can do from the comfort of your smartphone. Plus, with more people cobbling together incomes from multiple jobs with a variable pay, the task of managing cash flow is increasingly complex. Consumers want help, and they are beginning to expect help from their financial providers. In a recent J.D. Powers study, for instance, more than 75 percent of retail bank customers surveyed said they were interested in receiving advice from their bank, on topics including investments, how to improve their financial situation, retirement, and budgeting. The newest wave of innovation is focused on knitting these niche functions into a unified experience. Getting there will require an even broader window into people’s financial lives and more sophisticated algorithms to match the variety and complexity of people’s financial lives.
Collaborative solutions built on shared datasets will radically increase the accuracy, timeliness, and performance of non-competitive functions, creating mutual efficiencies in operations and improving the safety of the financial system. The physics metaphor – a weakening of “the bonds that have historically held together financial institutions” and the creation of “new centers of gravity where new and old capabilities are being combined in unexpected ways” – leads to several key findings of the financial services ecosystem's changing dynamics. These include the growth of collective solutions for shared problems and their consequences; the rise of uneasy data alliances; and the growing power of data regulators and their inevitable impact on the use of AI. Collective solutions will arise to fulfill the relentless need for data sets that can be fed into AI platforms, facilitating pattern recognition and problem-solving in, for instance, anti-fraud and anti-money-laundering (AML). Examples are the SWIFT messaging network and Early Warning Services – both bank-owned utilities – which are applying AI in real-time payment fraud monitoring. Such shared solutions can increase the accuracy, timeliness, and performance in non-competitive areas, spreading operational cost savings while improving the overall safety of the financial system. Almost any compliance-related activity that is not a profit-driver can be ripe for either process or data sharing. Nevertheless, you have to put a lot more controls in place to make sure that such data is not used by competitors or is attributable in ways that might destroy your competitive advantage.
As AI reduces search and comparison costs for customers, firm structures will be pushed to market extremes, amplifying the returns for large-scale players and creating new opportunities for niche and agile innovators. Artificial Intelligence is necessitating changing organizational structures and the creation of new roles, just as the internet did post-Netscape. Like the internet, Artificial Intelligence, once again, will change how business is conducted, and who conducts business. Initially, we will see the integration (or destruction) of traditional business and technology silos to allow the cooperation and data aggregation that is critical to running AI programs. AI models are, in some ways, like house plants. You need to care for them to keep them growing and thriving. They require continuous data collection, training, engineering, and monitoring to ensure their performance increases in accuracy and does not decay. New AI projects need close alignment to the challenges of the business and means to measure success. This all becomes a big challenge when you run multiple AI models, which forces data teams, technical teams, and business teams to all work together to ensure success. The future of AI-driven organizations includes the birth of the AI Center of Excellence (CoE), a new department that will be business-led and follow the growth levers defined by the corporate strategy. The CoE will own, consolidate and govern all corporate data assets for ready access to any project or organization that needs to train models. From a business standpoint, artificial intelligence programs at scale require tight alignment with core objectives. Machine learning models often provide the missing link between data, analytics, and measurable outcomes. Roles like Chief AI Officer, AI Strategist, and AI Product Manager will emerge to ensure data collection and AI uses case identification all tie back to the corporate strategy. These new roles make sure success is well-defined and prioritize projects that require the least amount of resources with the maximum return on investment. Technology, data, and DevOps are a few of the most important components of AI-driven organizations. New technologies will be acquired to capture, store, access, and process data. Models require a tremendous amount of data to perform accurately. And since ‘data is the new oil’, savvy organizations are hoarding data produced by everyone — and everything — from consumers to sensors to leverage for machine learning.
In an ecosystem where every institution is vying for diversity of data, managing partnerships with competitors and potential competitors will be critical but fraught with strategic and operational risks. No single organization can master all of AI. It’s too big. It’s a worldwide ecosystem of brilliant minds and spectacular tools, scattered through tens of thousands of companies and universities. A university-industry-government partnership is seeking to recruit talented researchers to advance AI research and meet industrial needs. From robots to facial recognition, artificial intelligence (AI) has become part of everyday life and is reshaping the workplace. As part of China’s push to advance its high-tech industries, in 2017, the state council launched the Next Generation Artificial Intelligence Development Plan in mid-2017, outlining a national ambition to become a leading AI power by 2030. Soon after guidelines were established, Zhejiang Lab was founded in Hangzhou. An initiative by the Zhejiang provincial government has seen Zhejiang University join forces with China’s e-commerce giant, Alibaba Group. With 30 billion RMB in government funding, Zhejiang Lab was set up to meet the industry’s AI needs. It aims to deliver original and innovative research in intelligent perception, computation, network, and systems through major projects and building big science facilities. To facilitate the future of AI, Zhejiang Lab has recently kicked off a global recruitment drive to attract bright minds. It is looking for researchers, and chief scientists in machine learning, open-source AI platforms, AI chips, cognitive computing, robotics and automation, big data, human-machine collaboration, intelligent network, and intelligent perception.
Regulations governing the privacy and portability of data will shape the relative ability of financial and non-financial institutions to deploy AI, thus becoming as important as traditional regulations to the competitive positioning of firms. Artificial intelligence applications in RegTech are in their infancy today although regulatory compliance is ripe for automation through AI, as evidenced by the emergence of many startups and AI vendors in the space. Also, the major applications today seem to be around using predictive analytics to stress test a company’s business performance under different market conditions, automatically tracking compliance requirements and establishing an email monitoring and filtering systems. Data security regulations have been steadily getting more strict and with the recent GDPR mandate in the EU, businesses can expect that data security norms might get tighter in the future. Last but not least, AI use-cases in RegTech are still few in number and the next two to five years might see the emergence of many established AI applications – just as was seen for AI applications FinTech.
Talent transformation will be the most challenging speed limit on institutions’ implementations of AI, putting at risk the competitive positioning of firms and geographies that fail to effectively transition talent alongside technology. As technology, including robots, artificial intelligence, machine learning, and other forces change the nature of work, employees will need new skills to adapt to shifting roles. Research firm Gartner predicts that employees who regularly update their skill sets and invest in new training will be more valued than those with experience or tenure. But it’s not going to be easy. The World Economic Forum’s “Future of Jobs 2018” report estimates that, by 2022, more than half (54%) of employees will require significant skills updating or retraining. More than one-third (35%) will need about six months to get up to speed, while nearly one in five will require a year or more of additional training. And employers might not be much help. A 2019 global survey of employers by consulting firm Deloitte found that 86% of respondents rated the need to improve learning and development (L&D) as “important” or “very important.” But just 10% felt ready to “very ready” to address that need. As digital transformation affects so many businesses, a 2018 Gartner report found that just 20% of employees have the skills they need for their jobs now and in the future. In addition to technology, digital, and data acumen, soft skills are also going to be increasingly in demand. As workplace environments experience fast-paced change and computers add a more straightforward, just-the-facts element to work, the ability to communicate, collaborate, and effectively work with others will be essential.
AI will necessitate a collaborative re-examination of principles and supervisory techniques to address the ethical gray areas and regulatory uncertainties that reduce institutions’ willingness to adopt more transformative AI capabilities. From 1927 to 2019 there have been more than 100 films produced worldwide about artificial intelligence. And while some scenarios are depicted in a good light, the rest are downright horrific. In movies such as The Terminator, The Matrix, Avengers: Age of Ultron and many others, the movie industry placed into our shared imagination scenes demonstrating how more intelligent machines will take over the world and enslave or totally wipe humanity from existence. The potential for AIs to become more superior to any human intelligence paints a dark future for humanity. More recently, countries all over the world have entered the race to develop artificial intelligence with 20 countries in the EU releasing their strategies on AI development in both R&D and education. Artificial intelligence is red hot. But what ethical and practical issues should we consider while moving full-steam ahead in embracing AI technology? In our shared goal to transform business sectors using machine intelligence, what risks and responsibilities should innovators consider? Ethical considerations in the AI space:
Yes, the thought of increasingly present AI systems that surpass human intelligence is scary. And the ethical issues that come with AI adoption are complex. The key will be to keep these issues in mind in order to analyze the broader societal issues at play. Whether AI is good or bad can be examined from many different angles with no one theory or framework being the best. We need to keep learning and stay informed in order to make good decisions for our future.
Predictions for the soon-to-come AI applications in financial services are a hot topic these days but one thing is for sure: AI is rapidly reshaping the business landscape of the financial industry. There are high hopes for increased transactional and account security, especially as the adoption of blockchains and cryptocurrency expands. In turn, this might drastically reduce or eliminate transaction fees due to the lack of an intermediary. All kinds of digital assistants and apps will continue to perfect themselves thanks to cognitive computing. This will make managing personal finances exponentially easier, since the smart machines will be able to plan and execute short- and long-term tasks, from paying bills to preparing tax filings. We can also expect to see better customer care that uses sophisticated self-help VR systems, as natural-language processing advances and learns more from the expanding data pool of past experience. A new level of transparency will stem from more comprehensive and accurate know-your-client reporting and more thorough due-diligence checks, which now would be taking too many human work hours. As we can see, the benefits of AI in financial services are multiple and hard to ignore. According to Forbes, 65% of senior financial management expects positive changes from the use of AI in financial services.